Capstone - DeepApp
Introduction
As smartphones become inseparable from daily activities, users generate large amount of app usage data which can be collected by internet service providers. Such data contains pattern which could reflect user lifestyles and habits. From this, it is easy to see why being able to make accurate mobile application usage prediction, namely what apps will be used next, would be useful in improving both the user experience and smartphone hardware optimization.
Recently, the current state-of-the-art model DeepApp (Xia, 2020) has provided a general deep learning framework which could achieve context-aware predictions by using a multi-task learning architecture. It was also the first attempt to train a general model for multiple users and achieved state-of-the-art accuracy.
In this project, we adapt from DeepApp and propose several novel enhancements to improve the performance of the current state-of-the-art app usage prediction model. Firstly, we increased the contextual awareness of the model by adding spatial feature such as Point-of-Interest (PoI) distribution and weather feature. Secondly, we maximize the use of the available training data by including weekend data which was excluded during the training of the original DeepApp. Finally, we implement a different partitioning method in the training process to the “Tsinghua App Usage Dataset”. Results show that the enhanced model outperformed DeepApp in recall, precision, f1-score and AUC ranging from 7% to 11% despite having the access to less than one tenth of the original dataset, which was not available for the project.
Our model architecture
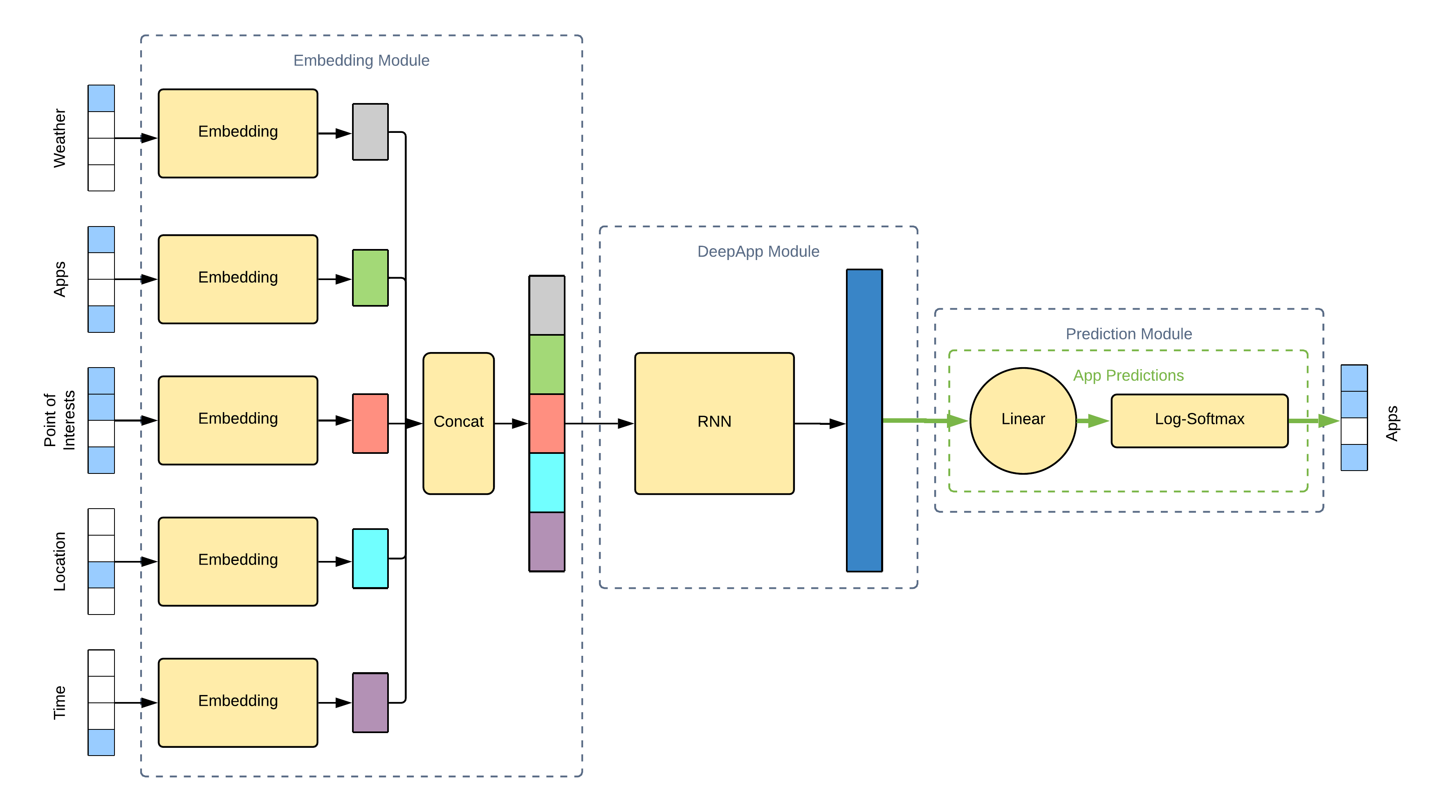
Original architecture
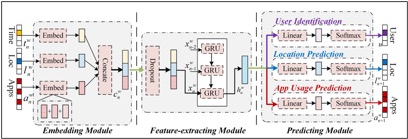
Credits
Supervisors:
Fellow group mates
Reference
Xia, T. a. (2020). DeepApp: Predicting Personalized Smartphone App Usage via Context-Aware Multi-Task Learning. ACM Trans. Intell. Syst. Technol..
Yu, D., Li, Y., Xu, F., Zhang, P., & Kostakos, V. Smartphone app usage prediction using points of interest. Proceedings of the ACM on Interactive, Mobile, Wearable and Ubiquitous Technologies (ACM IMWUT/UbiComp), 1(4), 174, 2018.